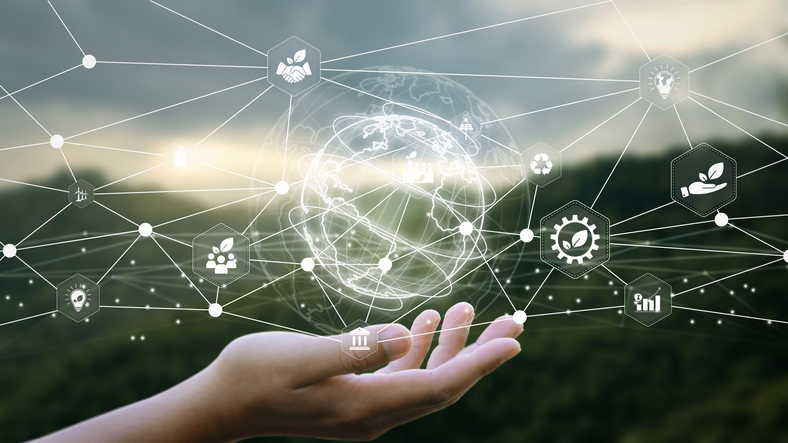
Discover insights from a senior CIO breakfast hosted by McGregor Boyall and Re-Use Technolog...
Discover insights from a senior CIO breakfast hosted by McGregor Boyall and Re-Use Technolog...
What's inside:A detailed market overview reflects 2024's challenges and sets e...
What's inside:A comprehensive market overview highlighting 2024's recruitment challe...
McGregor Boyall announces that after an incredible 15-year journey with the firm, Lucy Frost...